|
In the realm of data science, the significance of clean, reliable data cannot be overstated. Data cleaning, also known as data cleansing or data scrubbing, is a crucial step in the data preprocessing pipeline. It involves detecting and correcting errors, inconsistencies, and inaccuracies in the dataset to enhance its quality and ensure its suitability for analysis. Let’s delve into some essential data cleaning methods that every data scientist should master.
- Missing Value Imputation: Missing data is a common challenge in datasets and can significantly impact analysis outcomes. Imputation techniques such as mean imputation, median imputation, or predictive modeling can be employed to estimate missing values based on existing data patterns.
- Outlier Detection and Treatment: Outliers are data points that deviate significantly from the rest of the dataset. These anomalies can skew statistical analyses and machine learning models. Various methods like Z-score, IQR (Interquartile Range), or clustering-based approaches can help identify and handle outliers appropriately.
- Standardization and Normalization: Inconsistencies Chinese Overseas Australia Number in scale and distribution across features can impede model performance. Standardization (scaling to a mean of 0 and standard deviation of 1) and normalization (scaling to a range of 0 to 1) techniques ensure uniformity in feature scales, facilitating better model convergence and interpretation.
- Deduplication: Duplicates in datasets can distort analysis results and inflate statistical metrics. Identifying and removing duplicate records based on unique identifiers or similarity metrics is vital to maintain data integrity.
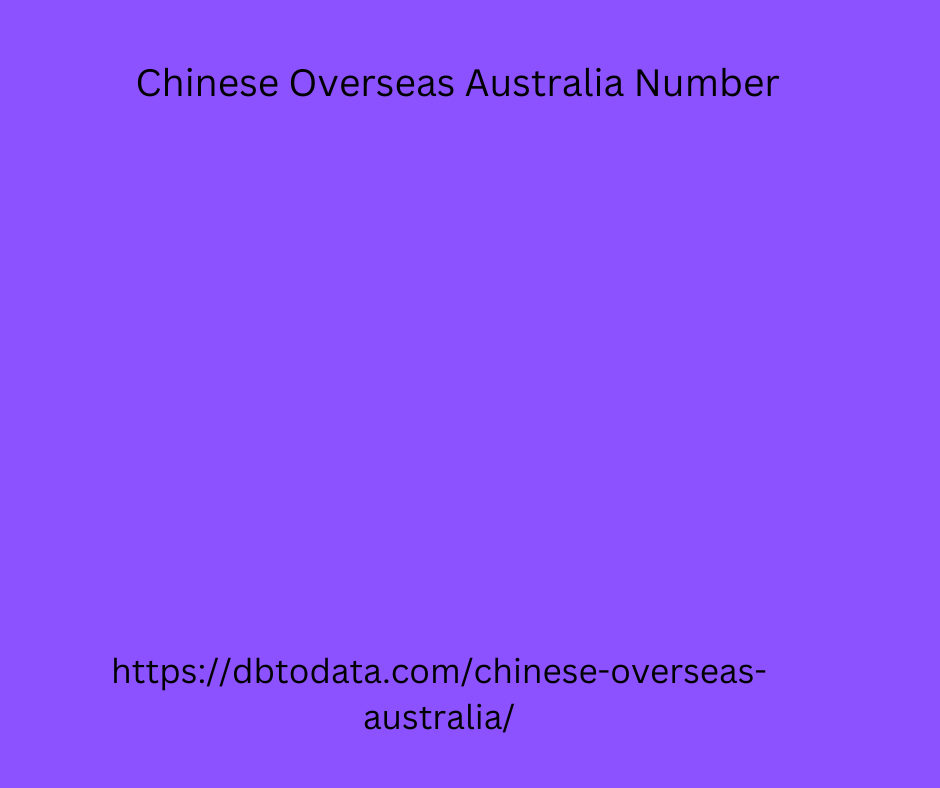
- such as lowercasing, punctuation removal, stop-word removal, and stemming or lemmatization can enhance the quality of textual data, making it more amenable to analysis.
- Error Correction: Data entry errors, typographical mistakes, and inconsistencies in formatting can compromise data quality. Automated tools or manual inspection coupled with domain knowledge can aid in detecting and rectifying such errors.
By mastering these fundamental data cleaning methods, data scientists can ensure that their analyses are built on a solid foundation of clean, reliable data, thereby fostering more accurate insights and decision-making.
|
|